by
The DermEngine Team on Mar 8, 2018
In recent years, big data has become a popular buzzword when talking about growth in the technology sector. However, there are confusions about what it is exactly, especially for people who are not involved directly in technical departments. This can be due to lack of leadership sophistication around the concept and its associated possibilities. Big Data: A Beginner’s Guide for Non-Technical People is a guide to address that. We will go through the core concepts and ideas behind Big Data and with the goal of keeping it jargon-free and simple.
1. Introduction
The concept of big data and the whole big data ecosystem are continuing to evolve with time and is a driving force behind a lot of latest technologies like artificial intelligence, machine learning, data science, deep learning, internet of things (IOT) and digital transformation.
In recent decades, with computers, internet, and smartphones becoming a common everyday commodity, we started generating a greater amount of data with every passing day. Today all of our actions have some kind of digital footprint which generates data (such as text messages, GPS, payment transactions, social media posts etc.). Sensor technologies in industries are other major sources of data being generated; the use of sensors in a typical factory increased ten fold in the last couple of years. Machines and devices in factories are equipped with all kinds of sensors nowadays which generates proportional data. All of this contributes to peta-bytes of data generated by our everyday lives.
2. What Is Big Data?
Big Data is the extremely large amount of data being generated by people, machines, and sensors which require new and scalable technologies to handle the data, as traditional systems were not designed to cope with the requirements associated with this new type of data. However, the amount of data is not the only reason for this name- the velocity of the data and the speed with which we need to process it are also reasons behind why we need scalable and distributable technologies.
The Four V's Of Big Data:
- Volume: The amount and scale of data being created every day is vast compared to traditional data sources we had in the past.
- Variety: The data comes from different sources in different structures and forms. Data is now being created not only by people but by machines, sensors etc.
- Velocity: The speed with which data is being generated and the speed with which we need this data to be processed. Data is generated extremely fast, the process that never stops even while we sleep. A single instance of high-velocity data is Twitter, where over 350,000 tweets are now sent worldwide per minute, equating to 500 million tweets per day.
- Veracity: Big Data is sourced from many different places. As a result we need to test the veracity/quality of the data.
3. What Can Big Data Do?
The amount of data we have now can be processed to find insights which were not even possible in the past. Some fields where Big Data is being used to help businesses are:
- Consumer Services: Makes everyday lives easier and more convenient. Examples include social media, e-commerce, gaming, financial transactions etc.
- Healthcare: Healthcare data-driven medicine involves analyzing vast number of medical records and images for patterns which can help the early detection of conditions, and aid in the development of clinical decision support tools.
- Space Exploration: NASA and other space organizations analyze millions and millions of data points to make models. These models are then used for the operations (like perfect environment) to land rovers on other planets and figure out atmosphere on other planets.
- Help In Disaster Management: Data especially the sensors data is and can be analyzed to predict where earthquakes are likely to strike next. Additionally, other data can be analyzed to prevent or to respond to natural as well as man-made disasters.
- Preventing Crime: Police forces across the world are increasingly adopting big data analysis to predict and implement strategies to deploy resources more efficiently and act as a deterrent where one is needed.
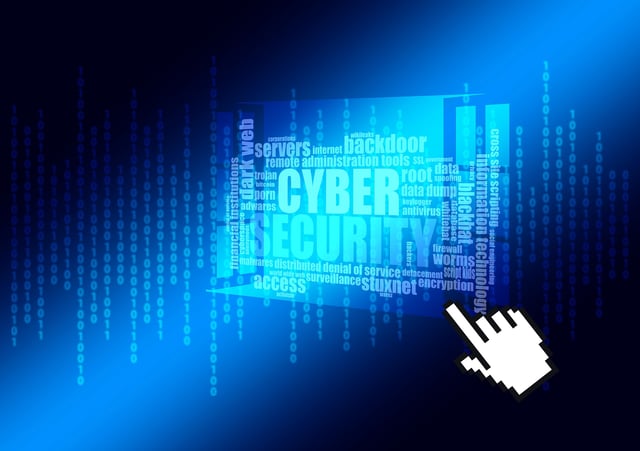
4. How does Big Data work?
Big Data is the major force behind data science. Data science works on the principle that more we know about anything and more data we have, we can analyze it and patterns will start to emerge.
All of the data that we need is available in structured, semi-structured as well as unstructured formats. This means that it cannot be easily converted into insights. Therefore, we need to clean, transform and structure all of this data based on the requirements. A large quantity of this data can also be in the format of pictures, videos, audio as well as multiple formats of text. To make sense of all this, big data projects often involve making use of the cutting edge technologies like machine learning. If we can teach computers how to process and make sense of the data using image recognition or natural language processing, computers can be more efficient in pattern detection than humans.
In order to process and store such large quantities of data, there must be appropriate resources to house and store this data. This need is what gave rise to organizations providing big data tools and stack through “as-a-service” platforms. Businesses can rent these services and use the storage and processing power from these platforms for big data and pay for the service. This model is making big data-driven discovery and transformations accessible to any organization and cuts out the need to spend vast sums on hardware, software, premises and technical elements.
5. What Are The Concerns Of Big Data?
With all of the advancements and insights big data provides us, it also comes with equal concerns:
- Data Privacy: With the increasing amount of digitization in our lives, a greater amount of data we share contains private data that we do not wish others to see. Due to this conflict, we as the consumer must find a balance between what we are comfortable sharing versus the personalized services provided by big data-driven companies and services.
- Data Security: With this data being shared across platforms and services, we can never be sure if our data is truly secure. Unfortunately, legal data protections laws still lack some details when it comes to personal data security. As such, companies continue to become the victims of hacks where large sets of customer data are compromised.
- Data Discrimination: The more data we share about our lives, the easier it is for organizations to discriminate people based on this information. For example, financial institutions already use these kinds of data to make decisions about credit services and loans. As the amount of available data increases, this type of scrutiny will naturally increase.
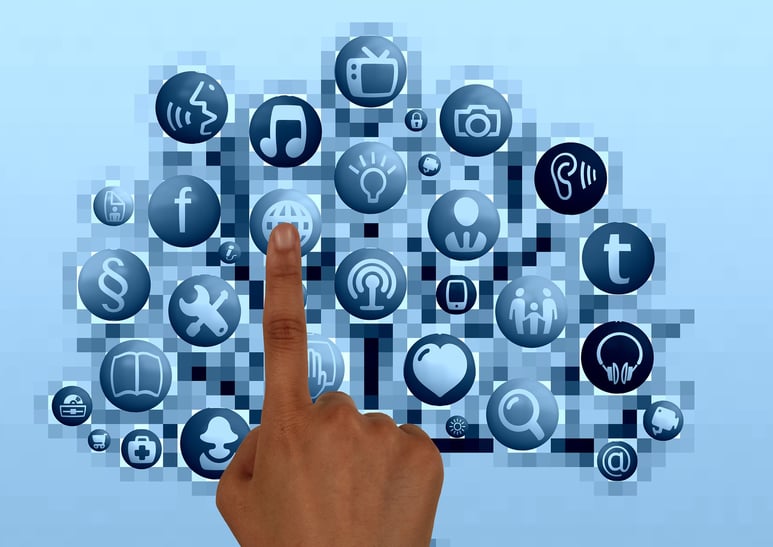
6. How Big Data Is Used In Data-Driven Organizations
Data-driven organizations working with big data tend to use Hadoop Stack. Hadoop is the most renowned and popular service for the Big Data Ecosystem. The Hadoop Stack which started with just Hadoop Map-Reduce and HDFS has expanded to include a wide variety of other technologies and frameworks. Three distinct scenarios how Big Data is being used in Enterprise Data Management are:
Big Data As An ETL And Filtering Platform: One of the biggest challenges with big data is extracting valuable information from a lot of noise filled data. Hadoop stack can read in the raw data, apply appropriate filters, implement required logic and output a summary or refined data set. This is what ETL is and moreover, this output data can further be used as an input layer for analysis, BI, reporting or even using more traditional systems like SAS.
Big Data As An Exploration Engine: Once the data is in big data cluster and is filtered and cleaned ready to be used, it can be added to the existing pile of analytics-ready data without having the need to re-index all the data again. This newly appended data along with the old one is always available to corporation to make use of.
Big Data Cluster As A Data Archive: Most of the historical data may not be available for use after a certain number of years. The traditional way of archiving data is with the use of tape or disk, but in case this data is needed for some reason it is extremely painful and time consuming to reload all data back in the storage for use. Big data clusters can change this, as the storage is comparatively cheap in distributed clusters which can allow us to keep all that data in the cluster without the need to archive and clean the cluster storage.
7. End Notes
When people and organizations initially started to hear about big data some years back, it was potentially dismissed as a fad or trend which would be quickly forgotten. However, it is clear that this has failed to be proven as such. In contrast, a wide variety of new technologies that are being developed are based on ideas created from big data and Hadoop. The amount of data available for use is increasing every day; it is time for organizations and people to take advantage of big data and use it to benefit their lives!
Interested in seeing how big data and artificial intelligence are being used towards enhancing the practice of medical professionals? sign up for a demo today!